The Internet Ethos in AI: Between Cloud and Edge
AI deployment choices matter. From cloud to edge, some organisations are reviving the Internet’s original ethos of openness and decentralisation—governing intelligence in ways that reclaim autonomy, privacy, and control.
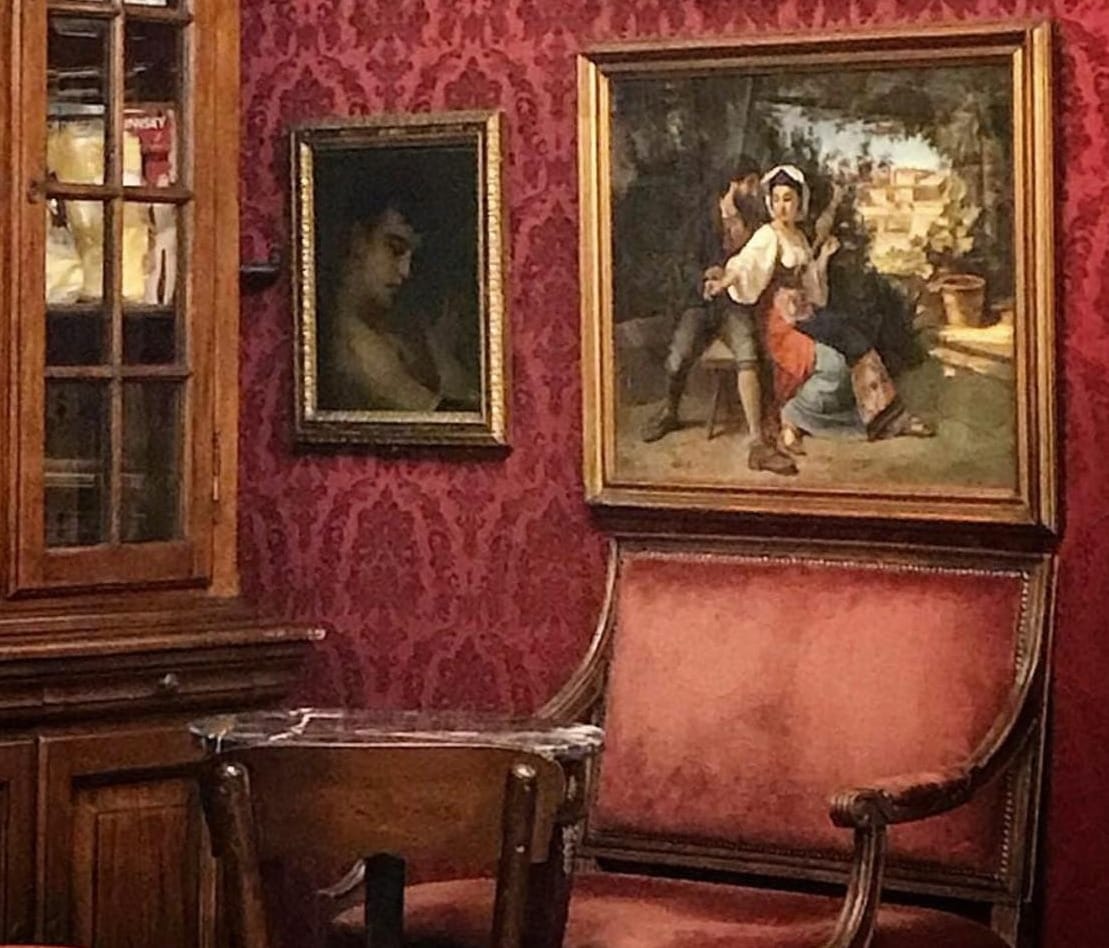
Amira, a PhD student, needed to keep her research private and under her control. After years building a valuable database, she wasn’t willing to upload it to the cloud. Instead, she bought GPUs—hardware optimised for running AI models—and built a personal assistant using open-source software. Her system helped her analyse data and support her applied research, all without relying on external services.
A small law firm faced a similar dilemma. To protect client confidentiality, it deployed an air-gapped legal AI agent powered by a large language model (LLM) hosted on internal servers. The system gave junior lawyers quick access to case law and helped senior partners draft contracts—while keeping all sensitive data in-house.
At a regional cancer clinic, doctors turned to an on-premises AI system built on retrieval-augmented generation (RAG), which paired a language model with a searchable medical library. It helped the team stay current with oncology research and guide treatment decisions—without exposing patient records to external platforms.
What unites these stories is a desire to benefit from AI while preserving autonomy. Whether safeguarding research, legal documents, or patient data, each example demonstrates how local AI deployments can deliver intelligence and efficiency —while upholding trust, privacy, and institutional independence.
But the choices behind these deployments — whether to adopt powerful AI tools hosted in the cloud or build and run them locally — reflect a deeper pattern, one we’ve seen before. In the early 2000s, the Internet was shaped by principles of openness, decentralisation, and user-driven intelligence at the network’s edges. By the 2010s, platforms and cloud services gained dominance, offering elastic scalability, always-on infrastructure, and managed complexity. Many organisations embraced these benefits, but often at the expense of autonomy and transparency.
Now, in the mid-2020s, AI is on a similar path. Cloud-hosted systems offer powerful capabilities: language processing, semantic search, and decision support, but also introduce dependencies. When adopted without oversight, these models may be trained on institutional data, influence outcomes, and operate within opaque, proprietary boundaries. The question is not just what AI can do, but whether it can operate with context, transparency, and reliability without undermining institutional autonomy.
As organisations navigate this new wave of digital transformation, key questions arise: Will AI work on their terms, or will they have to conform to the terms of external providers? Can concerns about security and privacy keep pace with AI’s rapid development? And are on-premises systems, air-gapped models, and self-governed AI stacks a practical path forward?
This post —the first in a series— explores the evolving landscape of AI deployment — from cloud platforms and hybrid setups to fully on-premises, air-gapped, and decentralised architectures. Each approach offers distinct advantages and trade-offs in terms of performance, control, security, and alignment with institutional values. Understanding these options is important not only for technical decision-making, but for shaping how AI supports human judgment, safeguards knowledge, and reflects the principles of the organisations it serves.
The AI Deployment Spectrum
Organizations face increasingly complex decisions about how to deploy AI systems, especially in sectors such as healthcare, law, and finance, where data sensitivity and regulatory compliance demand rigorous risk management. From hallucinations and bias to overconfidence in generative models, the challenges are not just technical — they are also ethical, legal, and strategic.
In response, organisations are adopting a spectrum of AI deployment strategies tailored to specific needs and risk profiles. Whether cloud-based, hybrid, on-premises, or decentralized, each architecture presents its own trade-offs, balancing enhanced human capability with the protection of institutional integrity.
Cloud-based AI services have seen an explosive boom, offering specialised capabilities ranging from text generation and image creation to analytics, and summarisation. These platforms are the most accessible and scalable, enabling rapid deployment and integration with existing systems. For many organisations, especially those lacking robust internal infrastructure, cloud services offer a fast path to AI adoption. However, the convenience often entails transmitting sensitive data to third-party servers — raising concerns about control, privacy, and compliance.
To address these concerns, major providers are becoming more adaptable. OpenAI’s GPT models support fine-tuning and policy controls through Microsoft Azure. There are plenty of options for Deepseek enterprise implementations. Google’s Vertex AI and AWS Bedrock offer customisable models with built-in encryption and governance safeguards. Anthropic’s Claude, while still externally hosted, emphasises transparency and safe behaviour, making it a growing choice for sensitive applications. As cloud-native platforms mature, they are becoming safer and more modular, remaining the most accessible entry point for AI adoption.
Hybrid AI deployments offer a practical middle ground — keeping sensitive data in-house while leveraging cloud resources for compute-intensive tasks. These setups are increasingly popular across industries seeking to balance performance, compliance, and autonomy. Zebra Technologies uses Envidia Run.ai to optimize GPU availability, while Verizon’s AI assistant — built with Google’s Gemini and trained on 15,000 internal documents — operates in a hybrid model that improved sales and customer service. Tools like Microsoft Azure AI Platform and Red Hat OpenShift AI extend cloud capabilities into private infrastructure. Platforms such as Stability AI and Perplexity Enterprise add further flexibility, enabling local deployment with optional cloud extensions. Hybrid models are particularly effective where data governance, custom workflows, and system integration are key priorities.
Fully on-premises, air-gapped, or decentralised AI deployments offer the highest levels of control, auditability, and security. Mistral AI, a European provider, offers open-weight models that can be fully self-hosted on edge devices or offline infrastructure, supporting encryption and integration into internal systems. Palantir and Anduril develop AI systems for secured environments: Palantir focuses on data fusion and decision support; Anduril, on autonomous systems for defence. At a more experimental level, projects like SingularityNET — a blockchain-based marketplace for federated AI agents — enable systems to learn from local data, collaborate independently, and operate offline, advancing privacy, autonomy, and ethical decentralisation.
There is no single “correct” deployment model. From cloud-native platforms to fully air-gapped systems, the AI deployment landscape offers a continuum of options, each with implications for trust, compliance, performance, and alignment with organisational values. The challenge is choosing an architecture that reflects not just technical needs, but institutional purpose and long-term vision. As AI continues to evolve, deployment choices will remain critical, not just in how AI performs, but in how it is governed, trusted, and integrated into human systems.
Conclusion
On a deeper level, these deployment choices rekindle the Internet’s original ethos: openness, decentralisation, and user empowerment. As AI becomes embedded in decision-making and institutional memory, it raises fundamental questions — not only about performance, but about alignment with the values and governance structures of the organisations it serves.
Can AI evolve into a trusted steward of institutional knowledge without becoming a gatekeeper of universal truth? Will isolated systems — however secure — risk fragmenting into epistemic silos, disconnected from broader dialogue? The future may depend on whether diverse, sovereign AI systems can coexist, interact, and learn from one another — preserving pluralism without sacrificing integrity, and reviving the spirit of a network built to connect, not control.
Future posts will examine the economics of AI deployment, how to prepare data for meaningful use, and the role of epistemic pluralism in shaping trustworthy AI systems. Together, these posts aim to support more deliberate, values-driven choices in how AI is integrated into the fabric of organisations.